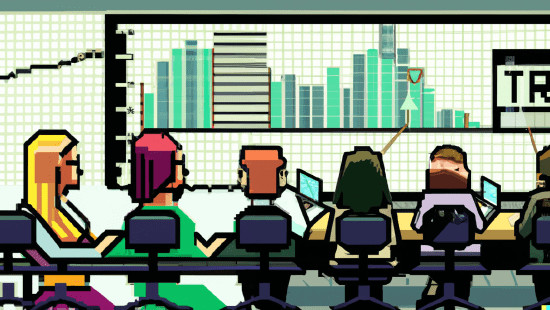
Foundations of Online Markets
Online market places are fundamentally impacting outcomes in the world. The services of technology companies like Google, Facebook, eBay, Netflix, Groupon, Amazon, and Uber are mediating the interaction between users who behave strategically. Ideas from game theory, economics, and computer science are fundamental for the proper working of these online markets. The Online Markets Lab studies foundational aspects of these market places with a focus on principles by which these market places can be designed to lead to more beneficial outcomes.
Research Areas

Mechanism Design for the Classroom
Mechanism design studies how the rules of a system can be designed so that good outcomes are obtained when individuals participating in the system are strategic. This project develops — and initiates the theoretical study of — a collection of mechanism design problems for the classroom. Specifically, it views the classroom as a computational system where some participants may manipulate the system to obtain better individual outcomes (i.e., the students) and some participants may be unreliable (i.e., the graders). The instructor aims to put in place policies with a number of natural objectives, e.g., optimizing learning outcomes, fairness of grading policies, and efficiency with respect to effort from participants (both students and graders). By understanding the classroom as an application domain for mechanism design, classroom outcomes can be improved. Moreover, a foundation for mechanism design that is grounded in practice can be established. This foundation may have an impact on other application domains for mechanism design, such as online markets.
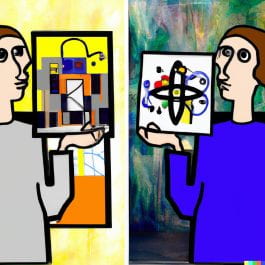
Benchmark Design and Prior-independent Optimization
Robustness is a key concern in mechanism design and a theory for robust mechanism design looks to identify mechanisms that perform well in many environments. This project considers two canonical frameworks for robust mechanism design: prior-free and prior-independent. Prior-free mechanism design compares a mechanism to a benchmark in worst case over inputs. Prior-independent mechanism design compares a mechanism to the optimal mechanisms (in expectation) in worst case over distributions of inputs. This project shows that for an optimized prior-free benchmark, the two frameworks give the same optimal mechanisms.
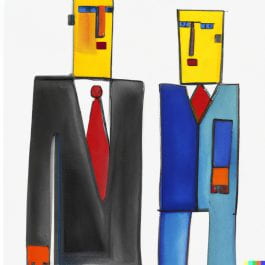
No-regret Foundations of Common-prior Mechanism Design
This project considers principal-agent problems where the Principal commits to a policy, the Agent takes an action, and Nature realizes a payoff relevant state. This problem abounds in economic theory including, for example, information design and contract theory. To understand behavior, economic theory imposes a common prior for the Principal and Agent about the state of Nature. This project replaces that common prior with online no-regret learning on the parts of the Principal and Agent. The Principal’s problem reduces to a robust version of the common prior problem.
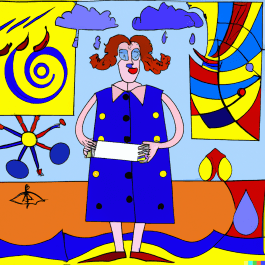
Optimization of Scoring Rules
Scoring rules enable a principal to elicit beliefs of a forecaster about a future probabilistic state, e.g., whether or not it will rain tomorrow. The forecaster reports her belief and upon realizing the state, the principal pays the agent according to the scoring rule.This project introduces an objective for optimizing proper scoring rules. The objective is to maximize the increase in payoff of a forecaster who exerts a binary level of effort to refine a posterior belief from a prior belief. In this framework we characterize optimal scoring rules in simple settings, give efficient algorithms for computing optimal scoring rules in complex settings, and identify simple scoring rules that are approximately optimal. In comparison, standard scoring rules in theory and practice — for example the quadratic rule, scoring rules for the expectation, and scoring rules for multiple tasks that are averages of single-task scoring rules — can be very far from optimal.