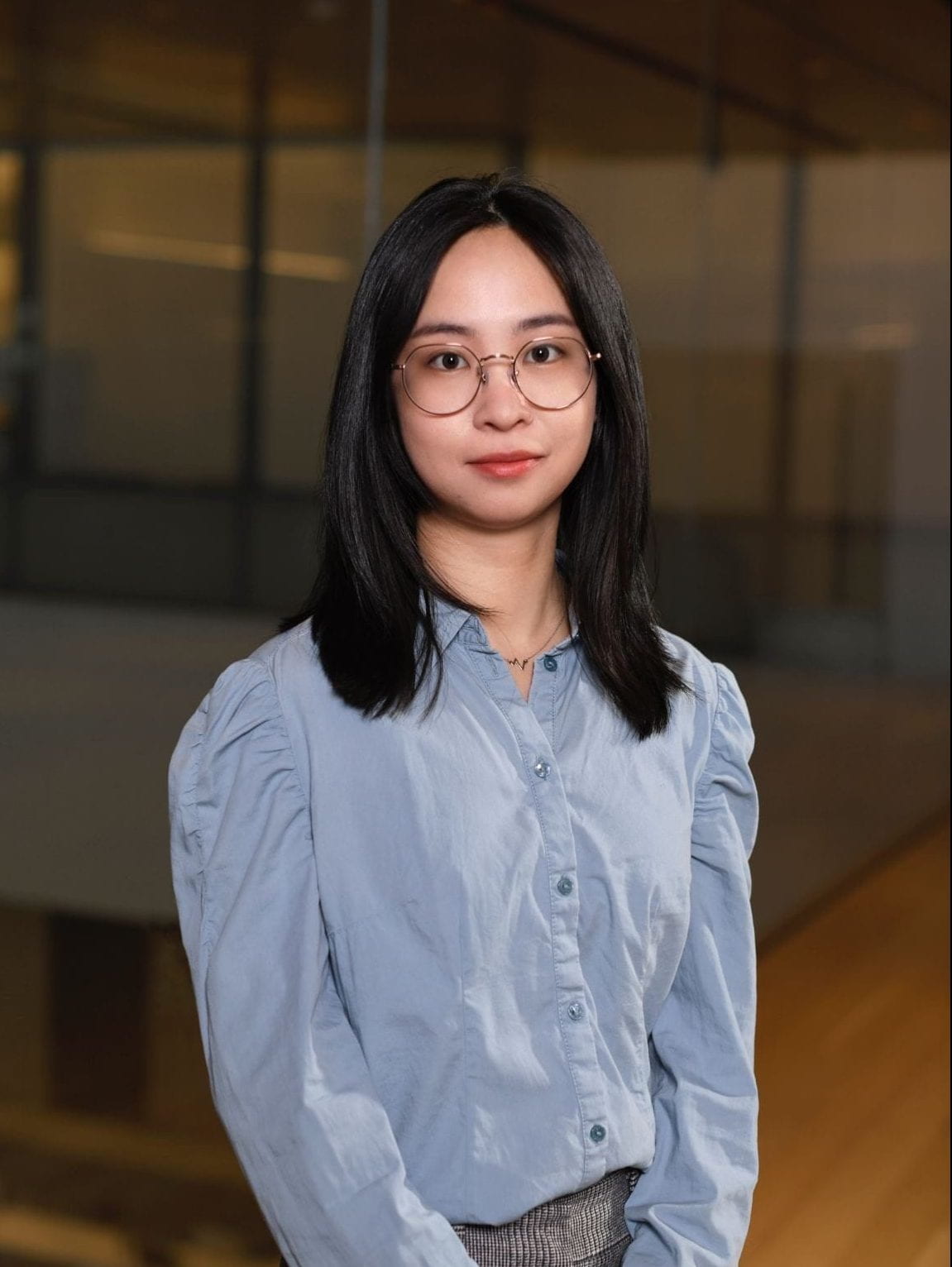
Bindan Zhang
Quantitative Marketing
Kellogg School of Management
Northwestern University
I am a Ph.D. candidate in Marketing at the Kellogg School of Management at Northwestern University. My research focuses on topics in the creator economy, influencer marketing, platforms, and recommender system.
I earned an M.S. in Applied Statistics from the University of Michigan, Ann Arbor in 2018. Prior to pursuing my graduate studies, I received a B.S. in Statistics from Sichuan University, China in 2015.
Working Paper
Creator Incentives and Influencer Economy (Job Market Paper)
The generation of platform content is essential for platform growth and competition. Typically, platforms incentivize participation and content creation through monetary rewards. Despite the explosive growth of social media platforms, there is little research on the effectiveness of creator incentives. I collect a novel panel dataset of creators with detailed video information from one of the largest video-sharing platforms in China. I examine the impact of a special type of incentive, namely the launch of an advertising platform that encourages brand-creator collaborations, on creator behavior and audience engagement. To account for self-selection, I combine a difference-in-differences approach with propensity score matching. I find that the launch of the advertising platform leads to a 25% increase in creator productivity but the increase does not lead to an increase in higher-quality content measured by audience engagements. Moreover, the increase in productivity is asymmetric across content categories with a positive association with the total number of ad-inserted videos in each category. By careful construction of treatment (ad-inserted) and control (non-ad) groups, with an assumption of a stable environment within a short time window, along with influencer panel data, I also find that ad insertion leads to a decrease in audience engagement for several engagement metrics. Moreover, well-established creators with more followers suffer more from the negative impact of ad insertion. This paper contributes to the literature on platform incentives and influencer marketing and provides insights for maintaining a healthy long-run ecosystem for creators, audiences, brands, and social media platforms.
Work in Progress
Influencer recommendation and brand relationship (with Jeremy Yang and Pankhuri Malhotra)
Selecting the right influencers is crucial for the success of influencer marketing campaigns. As a result, many platforms recommend influencers to brands based on their fit, follower count, and engagement rate. In this paper, we build a graph-based recommender system using Instagram data to investigate if an influencer’s past brand collaborations, or her brand portfolio, has additional predictive power of campaign performance in the future. We measure the relationship between brands along the dimensions of competition and image with brand bios and test two potential mechanisms. First, how does the number and variety of brands in the portfolio predict performance? Second, how does the relationship between the focal brand and brands in the portfolio predict performance? For practical implications, we discuss how platforms and brands can leverage influencers’ brand portfolios to improve the recommendation and selection, and how influencers can better manage their brand portfolios.
Maximizing enjoyment Over time on social media
In studying consumer satiation, researchers typically focus on the domain of household products or food and beverages. This paper explores users’ satiation behavior for social media content. The challenge of the task arises from using observational data collected under the recommender system. User behavior is confounded by the recommender system because the content provided to users is endogenous: contents are recommended based on the user’s previous interactions with the platform. This paper describes and utilizes exogenous variation steps from newly generated content to overcome the challenge.