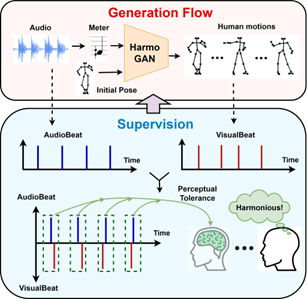
Recently, the cross-modal generation of audiovisual signals has gained much attention, with harmony being an essential element to consider. In this work, we propose a novel harmony-aware GAN framework with a harmony evaluation mechanism for improving the synchronization of the synthesized music-driven motion sequences. In the proposed evaluation mechanism, the accurate alignment between the cross-media beats is assured by refining the detected visual beats in a way to make them consistent with the mainstream spectrum-based audio beats, and simulating human attention by applying a saliency weighting mechanism. Incorporating such a mechanism, HarmoGAN is designed to be driven by beats based on musical meters. Apart from the traditional pose and GAN losses, the evaluation mechanism is also introduced in a hybrid loss function to act as a weakly-supervised harmony regularizer in the learning process. Accordingly, based on the given audio sequences, our model can generate harmonious human motions with a relatively small training dataset. The experimental results show that our proposed model significantly outperforms the state-of-the-art methods in terms of audio-visual harmony, both quantitatively and qualitatively. Some live results are also shown in the supplementary video https://youtu.be/4a7jCPtxmyE.