
This project is to develop a technology that enables automatic, continuous daytime data collection on bird interactions with solar energy facility infrastructure using computer vision that integrates machine/deep learning (ML/DL) algorithms and a high-definition, edge-computing camera.
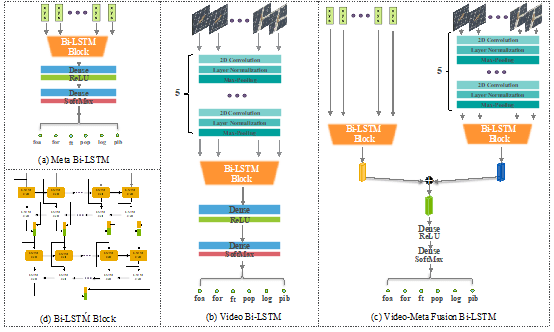
We develop a recurrent neural network-based model to automatically classify six avian activities around solar energy facilities. Our proposed model integrates critical feature engineering metadata with video frame data, enabling improved learning and more accurate activity classification. Our proposed Video-Meta Fusion Bi-LSTM model has been designed to classify six bird activities leveraging both the video data and additional engineered features (meta-data). we address the challenge of data imbalance during training and demonstrate the efficacy of our model in detecting and classifying different activities within video tracks. Additionally, we analyze the saliency/backpropagation map of the trained proposed model and validate its decision-making rationale.